SUBPROJECT 2:
Voice Restoration with
Brain Computer Interfaces.
One of the most essential human skills, our ability to speak, can be affected by traumatic injuries or neurodegenerative diseases such as amyotrophic lateral sclerosis, a disease that is expected to increase globally by 69% between 2015 and 2040 due to aging of the population and the improvement of public health. As this disease progresses, people who suffer from it stop being able to communicate verbally and require the use of devices that rely on non-verbal signals to communicate. Ultimately, some of these diseases can leave the individual in a state known as locked-in syndrome, in which the individual’s cognitive abilities are intact but the individual cannot move or communicate verbally due to complete paralysis of almost all voluntary muscles of the body.
In this project we intend to investigate the use of Silent Speech Interfaces (SSI) to restore verbal communication for these people. Silent Speech Interfaces are devices that capture non-acoustic biological signals generated during the voice production process and use them to decipher the words that the individual wants to transmit. While SSIs have been investigated mainly in the context of automatic speech recognition, this project focuses on direct speech synthesis techniques, thus generating audible speech directly from these biosignals.
More specifically, this project aims to develop a neural prosthesis in which electrophysiological signals captured from the cerebral cortex by invasive methods (electrocorticography) will be used to decode speech. Previous works have demonstrated the viability of this proposal for the case of automatic voice recognition. In this proposal we want to go a step further and investigate the generation of voice directly from neural activity, which would enable instant voice synthesis. Furthermore, as a consequence of brain plasticity and acoustic feedback, there is also the possibility that users could learn to produce better speech with continued use of the prosthesis. To transform neural signals into speech we will use the latest advances in brain activity sensors, speech synthesis and deep learning techniques.
During the project, databases of neural activity and voice signals will be generated and made available to the research community. In addition, new deep learning techniques will be developed.
The project will be carried out with the collaboration of a panel of national and international experts in the fields of automatic learning and silent speech interfaces. As a result of this project, we hope to initiate an innovative research with the ultimate goal of having a real impact on the lives of those with serious communication problems, allowing them to restore or improve the way they communicate.
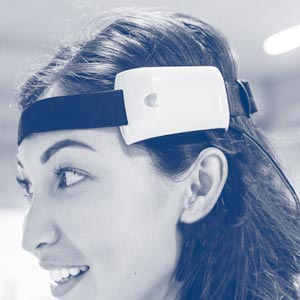
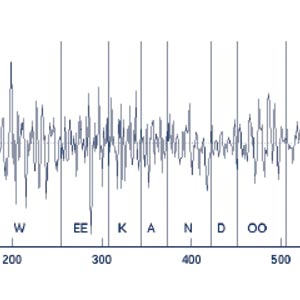
Objectives of the project
Coordinated project (SP1 + SP2)
- To explore the paths and advances in the application of state-of-the-art deep generative neural network architectures to improve the present quality and intelligibility of current SSIs using EMG and ECoG.
- To develop corpus, databases, protocols and best practices for research on SSI in Spanish language.
- To establish a new research line and, consequently, a research infrastructure for SSI in Spain.
- To strengthen the links between two of the most consolidated research groups on speech technologies at the national level: Aholab at UPV/EHU and SiGMAT at UGR.
Objectives for SP2
- Record the first large-scale data corpus in Spanish language with (a) parallel speech and intracranial neural recordings and (b) non-parallel recordings for imagined speech with only brain signals.
- Develop a high-quality baseline ECoG-to-speech system trained with parallel data recordings for synthesising continuous speech from recordings of the motor cortex neural activity.
- Investigate the use of transfer learning to adapt pre-trained DNN models trained on parallel data for the task of synthesise imagined speech.
- Investigate novel algorithms for DNN training with non-parallel data for the task of direct speech synthesis from imagined speech.